ASH 2017: Experts' Pick - Crowdsourcing For Improved Classification Of High Risk Myeloma Patients
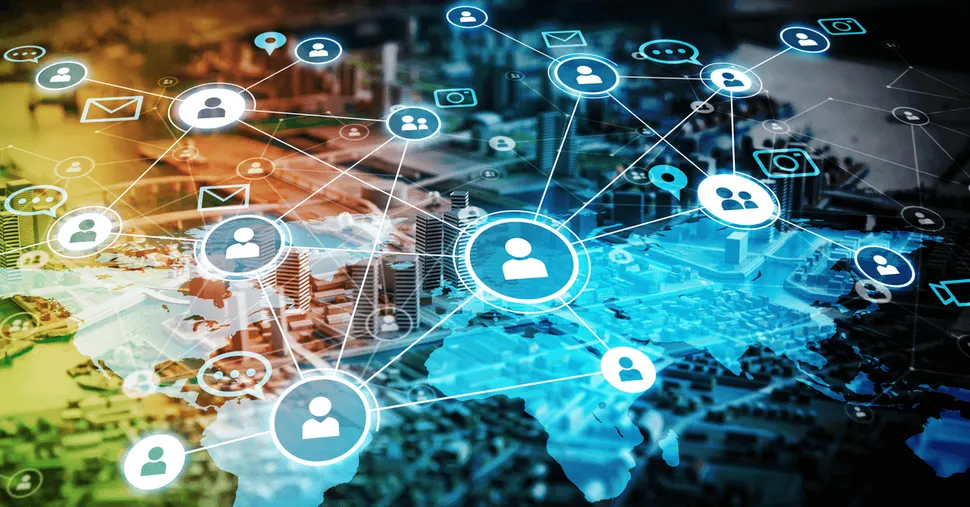
Many myeloma patients (defined as low risk) have shown excellent improvements in life expectancy. However, there is a subset of myeloma patients who rapidly progress or die within 18 months after diagnosis. Unfortunately, little has been developed or targets identified to improve the outcomes for these patients. Dr. Gareth Morgan has identified one abstract which combines a large multi-source database of myeloma patients and provides access to this data to worldwide researchers. All researchers using the data will help determine if there is a best way to identify and classify this subset of patients. This truly is a revolutionary way of taking what used to be one team at one location working on a solution, to having 290 teams from all over the world working in concert towards the same goal. This may be a game changer in the way we classify cancers and identify targets for future evaluation.
Dr. Morgan has chosen Abstract 265 as one of his favorites.
Abstract 265 - Crowdsourcing a High-Risk Classifier for Multiple Myeloma Patients
Dr. Gareth Morgan, M.D. FRCP, FRCPath, Ph.D, Professor of Medicine, is the Director of the Myeloma Institute at UAMS. He is also the deputy director of the Winthrop P. Rockefeller Cancer Institute at UAMS. Dr. Morgan came to UAMS from The Royal Marsden NHS Foundation Trust and The Institute of Cancer Research in London, where he was a Professor of Hematology and Director of the Myeloma Institute at UAMS, one the world’s best and most respected institutions for myeloma treatment. The Myeloma Institute has consistently produced some of the best survival rates and life expectancy of any of the world’s best myeloma facilities.
Dr. Morgan states, "Crowdsourcing can lever an unknown quantity of highly relevant experience to address significant clinical questions. In this case high risk disease will benefit from investigators addressing whether it can be recognised when patients first present to the clinic."Discussion, Results, and Conclusion
There is an urgent need for a precise risk stratification model to identify this subset of patients to allow selection of alternative therapeutic approaches. Current risk stratification practice is based on plasma cell cytogenetics and clinical stage at presentation, and, to a lesser degree, myeloma cell gene expression. The Myeloma Genome Project (MGP), an industry-academia collaborative project aims to develop highly accurate, clinically-implementable prognostic tests for MM patients. As part of the broader MGP effort, The MM DREAM (Dialogue for Reverse Engineering Assessments and Methods) challenge was conceived as an innovative approach to accelerate the development and evaluation of risk models in newly diagnosed MM. We collected large and diverse datasets from multiple collaborative groups and recruited a community of computational biologists, statisticians and leading experts in MM to build and test models (including the DREAM community of 20,000 solvers). Methods They assembled nine datasets comprised of 3,077 subjects, four data sets are publicly available and were provided for model training/development, while 5 data sets are previously unpublished and blinded to participants for model validation. The Challenge goal was to accurately predict which newly diagnosed myeloma patients would progress (or die) within 18 months of diagnosis. We challenged the community to identify high-risk patients using DNA-based, RNA-based, or a combination of RNA, DNA, cytogenetic and demographic features. As an additional incentive, $150,000 in prizes will be awarded to top performing teams. By the first month of the competition, 290 teams from around the world had signed up for the Challenge. We compare competitors’ performance against state of the art classifiers based on gene expression (e.g. UAMS-70, EMC-92), patient characteristics (e.g. International Staging System stage combined with age), and cytogenetics. These were used as a benchmark upon which winning models needed to improve. Results /Conclusion Based on implementation of several published benchmark models on a preliminary set of validation data, the top performing published DNA-based model was based on mutation burden, the top performing published RNA-based model was the UAMS-17, and the top performing clinical based model was a combination of age and the International Staging System (ISS). The unique combination of over 3,000 patients’ worth of molecular and clinical data allowed precise model generation. Top performing teams identified novel prognostic feature sets for high risk MM, establishing new benchmarks for the field, and final results from the top performing models will be presented. Such novel models will be integrated with the broader efforts within MGP for validation and clinical test development. The results of this effort demonstrate that data sharing coupled with the power of crowdsourcing can contribute to the development of robust and clinically-implementable models for risk-stratification of patients with MM. Thank You, Dr. Morgan for such an outstanding favorite abstract and for all you and your team do for the myeloma patient community. Myeloma Crowd initiated its research on the premise crowdsourcing and crowdfunding which could be used to fund high quality clinical trials, and it has been a resounding success. This abstract takes the "Wisdom of the Crowd" to new levels in the field of medicine.
Many myeloma patients (defined as low risk) have shown excellent improvements in life expectancy. However, there is a subset of myeloma patients who rapidly progress or die within 18 months after diagnosis. Unfortunately, little has been developed or targets identified to improve the outcomes for these patients. Dr. Gareth Morgan has identified one abstract which combines a large multi-source database of myeloma patients and provides access to this data to worldwide researchers. All researchers using the data will help determine if there is a best way to identify and classify this subset of patients. This truly is a revolutionary way of taking what used to be one team at one location working on a solution, to having 290 teams from all over the world working in concert towards the same goal. This may be a game changer in the way we classify cancers and identify targets for future evaluation.
Dr. Morgan has chosen Abstract 265 as one of his favorites.
Abstract 265 - Crowdsourcing a High-Risk Classifier for Multiple Myeloma Patients
Dr. Gareth Morgan, M.D. FRCP, FRCPath, Ph.D, Professor of Medicine, is the Director of the Myeloma Institute at UAMS. He is also the deputy director of the Winthrop P. Rockefeller Cancer Institute at UAMS. Dr. Morgan came to UAMS from The Royal Marsden NHS Foundation Trust and The Institute of Cancer Research in London, where he was a Professor of Hematology and Director of the Myeloma Institute at UAMS, one the world’s best and most respected institutions for myeloma treatment. The Myeloma Institute has consistently produced some of the best survival rates and life expectancy of any of the world’s best myeloma facilities.
Dr. Morgan states, "Crowdsourcing can lever an unknown quantity of highly relevant experience to address significant clinical questions. In this case high risk disease will benefit from investigators addressing whether it can be recognised when patients first present to the clinic."Discussion, Results, and Conclusion
There is an urgent need for a precise risk stratification model to identify this subset of patients to allow selection of alternative therapeutic approaches. Current risk stratification practice is based on plasma cell cytogenetics and clinical stage at presentation, and, to a lesser degree, myeloma cell gene expression. The Myeloma Genome Project (MGP), an industry-academia collaborative project aims to develop highly accurate, clinically-implementable prognostic tests for MM patients. As part of the broader MGP effort, The MM DREAM (Dialogue for Reverse Engineering Assessments and Methods) challenge was conceived as an innovative approach to accelerate the development and evaluation of risk models in newly diagnosed MM. We collected large and diverse datasets from multiple collaborative groups and recruited a community of computational biologists, statisticians and leading experts in MM to build and test models (including the DREAM community of 20,000 solvers). Methods They assembled nine datasets comprised of 3,077 subjects, four data sets are publicly available and were provided for model training/development, while 5 data sets are previously unpublished and blinded to participants for model validation. The Challenge goal was to accurately predict which newly diagnosed myeloma patients would progress (or die) within 18 months of diagnosis. We challenged the community to identify high-risk patients using DNA-based, RNA-based, or a combination of RNA, DNA, cytogenetic and demographic features. As an additional incentive, $150,000 in prizes will be awarded to top performing teams. By the first month of the competition, 290 teams from around the world had signed up for the Challenge. We compare competitors’ performance against state of the art classifiers based on gene expression (e.g. UAMS-70, EMC-92), patient characteristics (e.g. International Staging System stage combined with age), and cytogenetics. These were used as a benchmark upon which winning models needed to improve. Results /Conclusion Based on implementation of several published benchmark models on a preliminary set of validation data, the top performing published DNA-based model was based on mutation burden, the top performing published RNA-based model was the UAMS-17, and the top performing clinical based model was a combination of age and the International Staging System (ISS). The unique combination of over 3,000 patients’ worth of molecular and clinical data allowed precise model generation. Top performing teams identified novel prognostic feature sets for high risk MM, establishing new benchmarks for the field, and final results from the top performing models will be presented. Such novel models will be integrated with the broader efforts within MGP for validation and clinical test development. The results of this effort demonstrate that data sharing coupled with the power of crowdsourcing can contribute to the development of robust and clinically-implementable models for risk-stratification of patients with MM. Thank You, Dr. Morgan for such an outstanding favorite abstract and for all you and your team do for the myeloma patient community. Myeloma Crowd initiated its research on the premise crowdsourcing and crowdfunding which could be used to fund high quality clinical trials, and it has been a resounding success. This abstract takes the "Wisdom of the Crowd" to new levels in the field of medicine.

about the author
Gary Petersen
Gary is a myeloma survivor and patient advocate. His work centers around helping patients live longer by helping them to find facilities who are beating the average survival statistics. You can find Gary's site at www.myelomasurvival.com and follow him on Twitter at @grpetersen1
More on Treatment Advances
Trending Articles
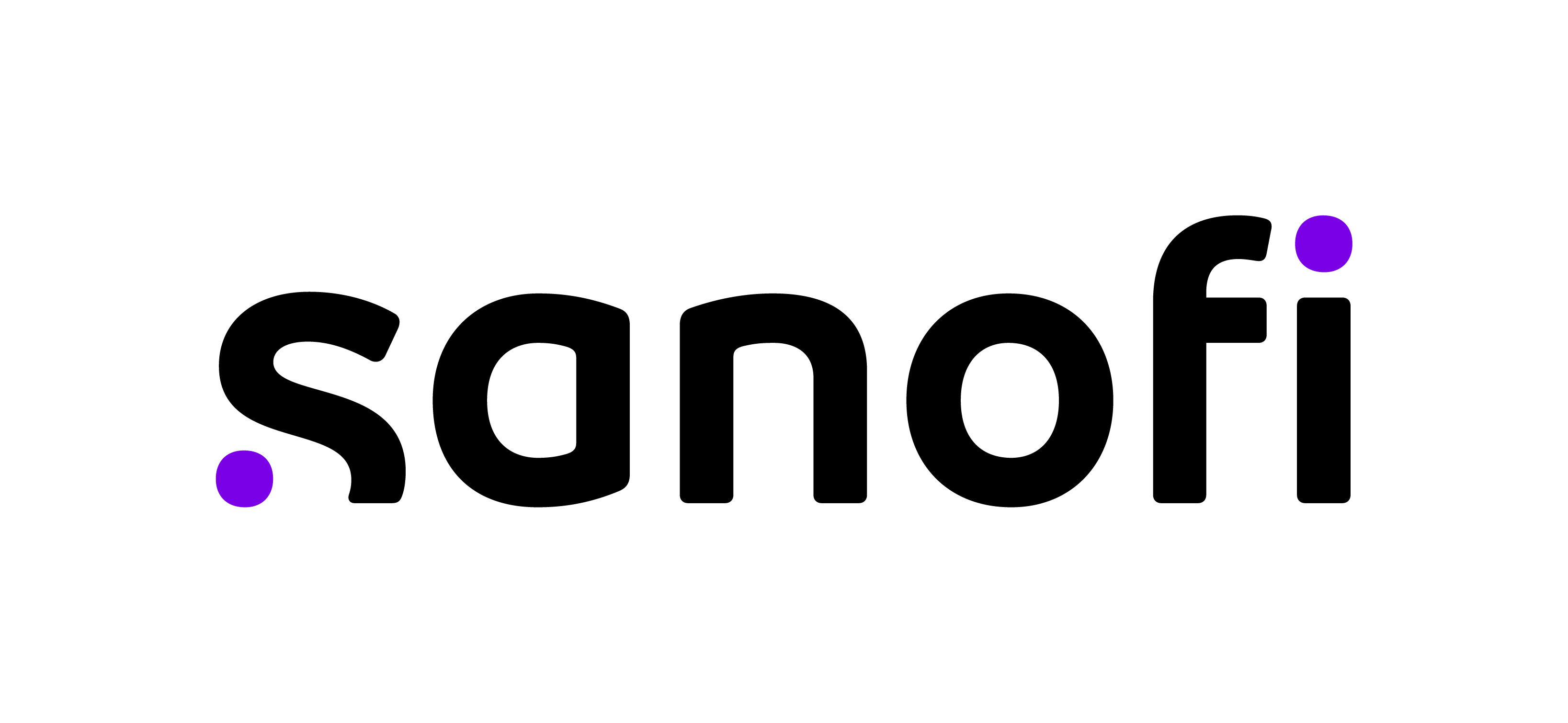
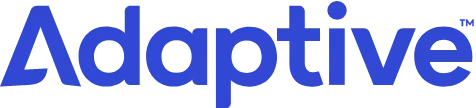
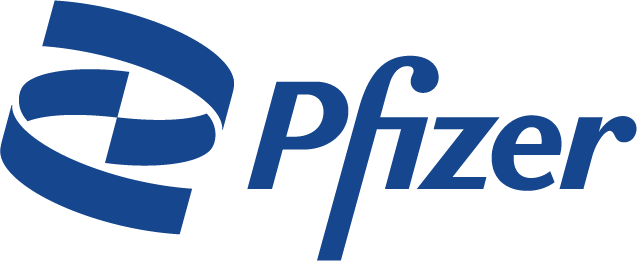

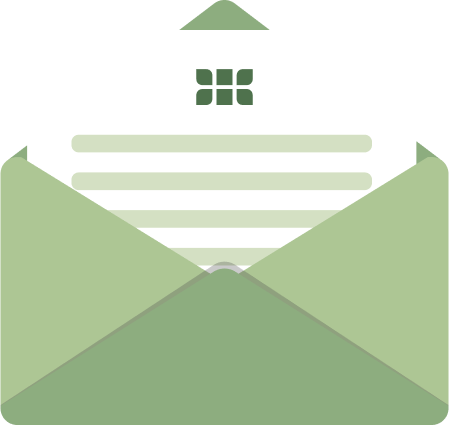
Get the Latest Multiple Myeloma Updates, Delivered to You.
By subscribing to the HealthTree newsletter, you'll receive the latest research, treatment updates, and expert insights to help you navigate your health.