Can Doctors Predict Response to Multiple Myeloma Therapy? A Team Effort to Do Just That with Dr. Brian Van Ness, PhD, University of Minnesota
Episode Summary
Why do some patients respond to treatment when others become resistant to common myeloma therapies? Dr. Brian Van Ness, PhD of the University of Minnesota shares his work in the lab and with the Mayo Clinic to dive deeper into the genetics of myeloma to determine which type of myeloma cells are responding to standard therapies and which are not. He compares the Human Genome Project, which cost $2-3 billion and took 11 years to finalize, to the current capacity now available to do the same work for $1,000 in less than a week. But he also shares the challenge: how do you sort through all of the information to find what could be relevant and important? Dr. Van Ness and the University of Minnesota have partnered with the Mayo Clinic to unite his laboratory capacity and expertise in tumor cell genome sequencing and modeling with the clinical practice at the Mayo Clinic. Their goal for this first project is to identify which myeloma tumors become resistant to proteasome inhibitors and which do not, allowing myeloma doctors to identify when this standard class of drugs will be most effective. With that valuable information doctors could predict response, prescribe secondary drugs to overcome the resistance, or stage their use to increase their effectiveness. Sorting through the mass of data will require teamwork from researchers, doctors and data analysts to make sense of the critical information that is now available. The day of myeloma genetics has arrived and is now headed to real-world clinical application. The Myeloma Crowd Radio Show with Dr. Brian Van Ness, PhD
Full Transcript
Jenny: Welcome to today's episode of Myeloma Crowd Radio, a show that connects patients with myeloma researchers. I'm your host, Jenny Ahlstrom. We'd like to give special thanks to our episode sponsor, Takeda Oncology, for their support of Myeloma Crowd Radio and myeloma patients. Now we recently concluded our series for the Myeloma Crowd Research Initiative or what we call the MCRI. If you haven't already listened to the shows, we invite you to educate yourself about these projects. They're specifically designed to address the needs of high-risk patients but are equally critical for all patients. This is the very first time that patients as a united group are helping to select and fund research that will make a real difference in our clinical outcomes and we invite you to join us. You can help us get ready by creating your own fundraising page on https://mcri.myelomacrowd.org. You can also check out our shop on Myeloma Crowd - all proceeds from these items go to fund this new initiative. We will be announcing the selected projects shortly and then we'll launch a patient-driven fundraising campaign for them. As Gary Petersen says in our video, this is a way that patients can help cure themselves. Now today we are very happy to have with us Dr. Brian Van Ness of the University of Minnesota. Dr. Van Ness spoke at Pat's Myeloma Beach Party in March in Florida and his research is where I believe myeloma is headed next, to precision medicine. He is learning much about treatment resistance; why some patients have resistance to treatment and others don't and what we can do about it. He did such an excellent job that I wanted him to help us all understand it. So welcome, Dr. Van Ness, and let me introduce you before we begin.
Dr. Van Ness: Well, thank you. Thank you so much for the invitation, my pleasure to be here.
Jenny: We're so happy you're here. Dr. Brian Van Ness is a professor in the Department of Genetics, Cell Biology and Development at the University of Minnesota Medical School. He served as the Head of the Department for nine years then served as a Director of the Institute of Human Genetics and Director of a newly established Division of Medical Genomics. After receiving a bachelor's degree in biology and a master's degree in chemistry, Dr. Van Ness obtained his PhD in biochemistry from the University of Minnesota. He finished his postdoctoral work in molecular immunology with the Institute for Cancer Research in Fox Chase, Pennsylvania. His current research focuses on exploring how genetic variations influence disease progression and therapeutic response, particularly in cancer. He has published over 150 papers on his research studies. He is also Founder and CEO of a new company, Target Genomics, that focuses on guiding healthcare providers on the use of genomics. This centers on a searchable resource for identifying and implementing clinical applications of genetics in drug therapies. He was a Senior Fellow of Commercialization at Minneapolis-based Life Science Alley and the BioBusiness Alliance, and served on the Advisory Board for the Alley Institute. He has been active in regulatory and legal issues in genomics as an expert consultant in patent litigation and a member of an NIH supported committee, making recommendations on the return of genetic results. Dr. Van Ness has recently created a joint course with the Law School called “Genetics and Law.” In addition to teaching responsibilities, Dr. Van Ness is a frequent guest speaker for continuing medical education programs and patient support groups around the country on genomics and personalized medicine and specifically in myeloma. So thank you again for joining us.
Dr. Van Ness: Again, my pleasure and it was a real pleasure to have an invitation to meet patients and speak with patients at Pat's Beach Party. I think it was a wonderful venue. It should be a natural treasure that Pat puts this together and all the people that participated in that. It was a real thrill to be able to talk to people with a true vested interest.
Jenny: Well, we are certainly important stakeholders which is why we do this show and which is why we started this Myeloma Crowd Research Initiative because we really think patients need to start weighing in more than they can do today if we really want to have outcomes faster. So Dr. Van Ness, your presentation at the Beach Party was based around predictions of treatment response, how do you predict, so maybe you could start by reviewing just the challenges and problems today that exist in myeloma therapy.
Dr. Van Ness: Sure. I'm sure that all of my colleagues and patients agree that one of the challenges for cancer and particularly for myeloma is that there is an enormous amount of heterogeneity in how these tumors present. One person's myeloma is not necessarily the same as another person's myeloma. And as patients they get together and talk. We’ll agree that not everybody responds to the same therapy the same way - some respond well, some don't respond well and some have significant toxicities. So the challenge is to try to understand and identify which drugs are the best for which patients to try to increase and improve the effectiveness of the drug for that particular patient and to try to avoid some of the adverse or toxic side effects. So I think that's a major challenge for researchers and clinicians to identify the best therapies for each individual patient.
Jenny: And for patients themselves to select which protocol they'd like to go after as well, I would think.
Dr. Van Ness: I agree. As I talk to patient support groups, I am amazed at how incredibly educated the patients are, how knowledgeable they are and as a result of that, they should have a strong voice in making those kinds of decisions.
Jenny: Well, we agree also. So what makes myeloma patients so unique or various, I guess, might be a better word.
Dr. Van Ness: Well, one of the hallmarks of a lot of cancers is what we call genetic instability. For whatever reason we don't truly understand, the genome of cells that are normal cells that normally had normal function, starts having alterations. Pieces of DNA start moving around, DNA sequences starts changing and this creates a kind of instability in the cell and for many cells, that kind of instability will simply kill the cell. But when certain unstable components of the genome become reactivated or activated in different types of ways, the cells will do things we don't want them to do. They will proliferate when we don't want them to proliferate and they will go places we'd rather they not be. The problem with myeloma in particular is that kind of genetic instability can create lots of opportunities for cells to become malignant and proliferate. As I've talked to people and patient support groups, I might say if I want to get from point A to point B, if I want to go from here to Chicago, I can get to Chicago in a lot of different routes. The same is true with myeloma and many cancers. The ultimate progression of a cell and its inability to die and maybe respond or not respond -- there are a lot of ways to get there. And so as a result of that, both from the research and the clinical application, trying to understand better how each person's myeloma got to the point that it is and how it might respond becomes a particular challenge and it becomes one that can be addressed by understanding some of the genetic components of each person's tumor.
Jenny: And to go along with that, if you say the genetics of myeloma, do you have any indication at all what causes myeloma in the first place? I know there might be a variety of things that do but do you have any inkling about that at all?
Dr. Van Ness: Well, if I had the true answer, I'd be on lots of companies' panels or something. But what it comes down to is there's a lot of genes that when activated cause cells to proliferate. They are in fact proliferation genes. They are also genes that frequently put the breaks on cells that are altered. And so I think the best answer to give you is that we're recognizing that there are multiple ways and multiple genes and multiple pathways, all of which can result in this kind of malignant transformation. And that's particularly true in myeloma. There are certain cancers in which there seems to be a real defining genetic or altered protein in certain leukemias and lymphomas, for example. There are some very defining characteristics of their genetics. Not so much true with myeloma. There are common features that patients may share in terms of chromosomal abnormalities that one patient may share with another. But there are a lot of additional complexities to the genetics of every person's myeloma such that one particular event doesn't seem to be the hallmark of this particular disease. That's why this disease is particularly challenging to try to develop treatment strategies because there is that enormous amount of diversity.
Jenny: When we've talked to other researchers, it sounds like there are also primary triggers and then secondary triggers that might come later as well.
Dr. Van Ness: Well, that's true. Again, because of this instability, some of the primary triggers may be inducing proliferation. And then as the tumor continues to progress, you may be treating it with an effective drug and it may turn out that 90% of the tumor responds to that drug but 10% of the tumor generates potentially new genetic events which create resistance to that particular drug and then there's a selection process. 90% of the cells will die off but that 10% now becomes resistant and now progresses into what may be the relapse of a particular patient. We're trying to understand some of those sub-population dynamics that occur and that's some of the excitement of the most recent research that's going on is starting to get some pretty good insight as some of those early initiating events and then some of the later events that can occur in these sub-populations of cells. That becomes really kind of important because if you can understand the heterogeneity even within one person's tumor, that heterogeneity as you understand it may give you better insight as to what combination therapies might be most effective.
Jenny: And that gets pretty sophisticated so we are all unique. It just will require more education both on the doctor's side and then on the patient's side.
Dr. Van Ness: Yes, it does. And it does bring up this whole sort of the concept of where we started with the human genome project and where it all goes. This whole concept of the human genome project has become probably a familiar term to most people. In the early days, 20 years ago, human genome was probably a term some academics understood. But I would say that the public now is starting to understand a little bit more about genomes. The human genome project was a project that was initially devoted to providing the sequence of all of the component bases of DNA and there are three billion of these in every individual and every cell has this DNA and those three billion bases are lined up in a particular order which creates a code and that code is followed for every individual. And so a couple things that I think are worth considering in terms of the insight and the excitement that we feel about where we're going with this. In about 2001, the first human genome was published as a complete list of all of those bases in the genome, all three billion. Well, at that point, it took about 11 years to get that first genome. And people estimate that if you consider all the research teams that were working on getting that put together, the total cost was on the order of anywhere from $2 billion to $3 billion to get that first human genome. What has happened is that today, technology has progressed so incredibly fast that what took 11 years and $3 billion can now be done in about a week for about a thousand dollars. That is an incredible advance in technology. Now one of the things that came out of that was an appreciation that that first genome was simply representative. It was a representative genome of the three billion bases and the order of those bases. But the fact is as we've been talking about, it was representative. It doesn't represent everybody's genome because everybody's genome has some differences in the order of those bases which makes everybody unique. We are as a species, our human population, if you compare the DNA base sequence of one individual to another, they are about 99.9% identical. So all those three billion bases if you line them up and compare one person versus another, they are over 99% exactly the same. But if you consider that 1% difference, that's over a million differences in that DNA sequence. And as a result of that, everybody has their own sequence. The one place where you might have some significant identity well beyond that are obviously identical twins. But other than that, the human genome sequence is quite variable in every individual and what that means is that everybody's now going to have their own risk for developing diseases; they're going to have their own characteristics if they develop a disease. I don't care if it's a common cold or a malignancy. That variability is going to show up every single time on how their disease progresses and how they respond to therapy. Some people seldom get colds, some people get colds all the time, why is that? It's environmental exposure combined with sort of the genetic predispositions that we have. And so I think it's also important to point out that genetics is not the only driver of what we look like and how we might be at risk for diseases. Environment plays a huge part in that and trying to understand that interplay between our environmental exposures and our genetics is a huge field of exploration.
Jenny: Oh, and very deeply complex. Maybe you can relate this human genome project to myeloma. I read some time ago, I'm not sure when it was done, that the myeloma genome have been mapped. When did that happen and how did that happen and what does that even mean if we're all individuals?
Dr. Van Ness: So what was done and with a lot of support from the MMRF, there were a number of patient samples that were obtained and the tumor cells genome was sequenced. And one of the questions to ask is if you sequence the tumor genome and compare that sequence to the same patient's normal cells, what changes had occurred in the tumor at the DNA level that were different than the person's normal cells. And this was done probably about four years ago, some of these firsts publications were coming out where maybe 30 different myeloma genomes were sequenced. Well, what did we learn from that? What we learned that there were some recurring themes in terms, again, of genes that were altered or mutated or rearranged within myeloma cells. And as a result of that, if you take one of those myeloma tumor genomes, you could get the entire three billion base pair sequence of that myeloma. But I would hesitate to say that that represented THE myeloma genome. It represented one myeloma genome. But then if you compare it to the other 29 in that publication, there were differences among all of them. So I would say that yes, there has been a mapping of the myeloma genome and the appreciation, just like the human genome project, that there's a lot of variation in that genome sequence of one person's myeloma versus another. But it really does start getting to the heart of the absolute detail of the code that distinguishes a myeloma from a normal cell and one myeloma from another. And there's an enormous amount of data that's now being collected to increase the number of myelomas to try to get a really strong database of all those kinds of variations that may impact how a disease may progress and how it might respond to therapy. And that's kind of exciting. It's an exciting opportunity particularly since it's not going to cost $3 billion to do each one and it isn't going to take ten years to do each one. This can be done in relatively quick turnaround times for a relatively inexpensive amount of money compared to where we were ten years ago.
Jenny: And what do we do with that information? What do you do specifically because your expertise is in this area? So what does someone like you do with 29 or 30 samples of the genome?
Dr. Van Ness: What we are specific research has been asking a very specific question and that is can we identify a genetic signature or a code that would distinguish a tumor that responds to a drug therapy versus a tumor that doesn't. Or in our case, we are specifically going after those proteasome inhibitors like Bortezomib or Velcade, carfilzomib. So that class of drug has been a very effective therapeutic approach for many patients. The problem is some patients do not respond to that therapy very well and the other problem is for many patients that do respond to that therapy over a period of time, they develop a tumor that emerges that becomes resistant to that therapy. And we're trying to figure out can we identify those genetic signatures that will distinguish a responding tumor from a non-responding tumor. And we're doing it at a slightly different level of the genome. We're looking at gene expression. So now we're taking the DNA that now is expressed in the form of another component of the genome, the RNA, that then is made into protein. And we're looking at the expression of all of these genes and asking is there a variation in how these genes are expressed that distinguishes a responding tumor from a non-responding tumor. And we think we've identified some of those signatures. Now the question might be if a patient walks in, what's the clinical application of this? We are not at a point of clinically putting this into practice at this point because we have a lot of validation to do. But the idea would be that a patient might come into a clinic and have a profile done and that profile would say you're not going to respond real well to a proteasome inhibitor but you've got a profile that's going to respond really well to Thalidomide, a different class of drugs. So it might better distinguish which drugs are better for which patients. The other thing that we've worked on is the fact that we know that many clinical applications are multiple drugs and so if a person comes in with a profile that might have some level of resistance to a proteasome inhibitor, we, from the profile, are trying to figure out what might be a second drug that would then help you get beyond that kind of resistance. In other words, you might have some level of resistance to a proteasome inhibitor but if we add a second drug to it, the sensitivities come back again. And so we're trying to develop better predictors not only of which drug might be the best drug but which combinations of drugs might be best for each individual patient. That's a goal we're nowhere near of having that implemented in the clinic quite yet but given the power of genetics and the ability to do some pretty strong computational and computer programming modeling, I think we're reaching those points where some of these signatures, if you will, can actually be meaningful and clinically actionable when patients walk in the door. Whether they walk in the door with a new diagnosis or they walk back in the door with a tumor that now has become resistant to a therapy, I think some of these profiles could be very informative in instructing oncologists what might be the best initial therapy or in a case of a relapse, what might be the next best therapy on that. And I think in fact, one of the reasons you're seeing better responses and longer survivals in myeloma patients is because the number of drugs that are available to treat myeloma has increased and increased effectiveness. And I think the good news for patients is that there are some very effective therapies and even if those therapies might start developing some resistance, there are other therapies that then can do a good job of attacking the myeloma cells. So I think it's a particularly exciting time in new drug development and linking the new drug development to better predictors of how each individual patient is going to respond.
Jenny: And your combination therapy seem to be really where myeloma is always at and what you're saying is it might smack one part down and the other piece might help with the other and so together, they'll always be probably more powerful than ever having one alone. (therapies)
Dr. Van Ness: Yes. We frequently look at these drugs and refer the fact -- the term we use is can these drugs work synergistically? And by definition of synergistically, what it means is that will the combination of the drugs work better than simply the added effect of the two drugs. You'll actually get better than the added effect because each drug helps the other drug work even better. Just in a simple point, if one drug can kill 30% of the tumors and another drug can kill 30% of the tumor, the two drugs together might kill all of the tumors because they work together better than each one individually. Jenny: Okay, perfect. Would you like to explain the project that you have going on between the University of Minnesota and the Mayo Clinic? Just describe your project and what its aims are and a little more about it? Dr. Van Ness: Sure, let me just give a little background on these partnerships. The one thing the state of Minnesota recognized at the legislative level with some education from various clinical and academic communities was that there was really an opportunity to develop collaborative arrangements from two world-class institutions in the state of Minnesota. You've got the Mayo Clinic and you've got the University of Minnesota. And the state basically said, "You guys really should be working together." And there is a lot of collaboration between the two institutions but nothing helps collaboration like a pot of money. It sounds crude but there's a reality there. So the state said, if we put a pot of money and you guys can get together and have an application process and it becomes just like your crowdsourcing, it becomes a competitive application process and it's not restricted to anything other than getting people at the Mayo Clinic and people at the University to work together. There are a number of these proposals that are submitted, they are reviewed by outside reviewers and they select two or three of these to support each year. This past year, one of the ones that they chose to support was a partnership between the Myeloma Group at the Mayo Clinic and our laboratory here. What we hope is this synergy between the research component is that our laboratory does a lot of modeling of these tumor cells in the laboratory. So we take tumor cells in the laboratory, we treat them with different drugs, we isolate DNA, we isolate RNA, we develop these kinds of signatures in the laboratory. And as we do that, we start predicting in our laboratory which cells in the laboratory might respond and which ones might not. The advantage of that is that we can turn these kinds of projects around a little more quickly than having to work with individual patients and we can experiment a lot more. The downside of that is that if it works in a cell in the laboratory, how do we know that we'll have any impact on a patient and that's where the partnership comes in. and although we see patients with myeloma certainly at the University of Minnesota, one would never argue that Mayo Clinic has a very strong clinical process in the therapeutic applications in myeloma. So the partnership has developed where we are developing these kinds of genetic signatures and then Mayo is going to apply these signatures to their patient samples to see if our signatures that we feel are predictive of response in our laboratory are actually going to be predictive response in their patients. So they're now going to take the patient samples and isolate the DNA and the RNA and develop these expression profiles and then ask whether our targeted signatures that we identify in the laboratory are in fact predictive of the patient outcomes at Mayo Clinic. If they are, then they potentially could be used in making therapeutic decisions but our research project is to first demonstrate whether we can make those kinds of predictions. The second part of the study is to ask the question when a patient comes in with a newly-diagnosed disease, is there already existing sub-populations in that tumor that might generate drug-resistant relapse so that if we can take our signature and look at one individual cell at a time in a patient sample, we might be able to identify sub-populations of cells that may respond or not respond. The new technologies are now allowing us to generate these kinds of genetic signatures one cell at a time and that's pretty exciting. So we're going to now apply that as well to some of the patient samples at the Mayo Clinic.
Jenny: Do you go backwards and look at anything retrospectively to say okay, the Mayo Clinic has all these myeloma patients and these are their genetic profiles and then you match it up with the laboratory work that you're doing or is it all just in the study as you go along?
Dr. Van Ness: I'm glad you brought that up. A lot of the initial studies we're doing are in fact retrospective looks which is one of the advantages, again, working with Mayo because Mayo does a very nice job of banking samples and making samples available that they already know the clinical history of the patient. So that makes doing this a whole lot quicker because we can ask the question not starting today do we have to wait three or four years to see if a patient is going to relapse, we have a database that's already existing. They have samples in their biobanks from such patients and that's some of our starting material that we're working with Mayo. So in fact, we are doing past analysis to see if our predictions will hold up.
Jenny: Right. It just seems to me this is a great partnership that could help speed things up by years.
Dr. Van Ness: I'm hopeful. It takes a lot of validation. You get something you think works really well and then you find out that it works well but only works well in 60% of the patients and then you have to scratch your head and say well, how do we get the percentage up higher so that it is actually worth doing? It's kind of iterative repeated process of testing, evaluating, redesigning and retesting and re-evaluating to the point where you get the best predictors you possibly can get. And that unfortunately does take time because you got to have that process has to continue in a sort of a cycle over and over again.
Jenny: So how long is your project?
Dr. Van Ness: Well, the state has given us two years. The state said, "Let's figure out something. We want to see something in two years." And one of the conditions of the proposals that everybody sent in was you had to demonstrate in the proposal that your outcome would have something that would provide clinical utility. In other words, not give me just the research answer that might be of scientific interest but give us something that actually could be applied in the clinic. And so that was part of the evaluation is to whether they thought we could after two years have something that could be applied in the clinic. And so that's part of our challenge right now is to come through with that promise in the proposal that we might have something that actually could be applied in the clinic.
Jenny: And then how far into this? Are you just beginning?
Dr. Van Ness: Yes, we're just in the beginning of this. We got the funding in place just around February or March so we're only few months into this right now. And we handle this, and again, technology works really well. The colleagues at Mayo include Vince Rajkumar and Shaji Kumar plus some of their bioinformatics people and genomics people down there and we meet periodically at least once a month by ITV. So we all going to a conference room and we can see each other and we can present data to each other without necessarily having to drive to each other's places because we can see each other and meet with each other in real time with the new technologies that we both have available.
Jenny: Sounds like a great project. Can you explain the heat map? In your presentation at the Beach Party, you had some diagrams. And I think what I'll do is take maybe a slide of that and put it inside of the show notes for today once we have the final post. So you can explain as if we were looking at it, I guess.
Dr. Van Ness: A heat map is the consequence of the computer generating a visual display of how the genes are being expressed. And what it does is it says, okay, I'm going to line up and imagine I'll have 50 genes. So I'm going to look at 50 genes and how they're expressed. And let's say that across every row, I'm going to have an individual tumor from 20 people. So I've now got 20 people who I'm looking at the expression of 50 genes. And what the computer will do is it actually color code and it will say if the gene is highly expressed, I'm going to color it red. If it's not expressed much at all, I'm going to color it black. And if it's actually decreasing in expression relative to normal say, I'm going to color it green. And what it does is it clusters the patterns of the genes that are going up and going down in individual patient's tumors. Now the way I presented it at the Beach Party is you end up with this bar code looking kind of thing that's a visual and what I presented at the Beach Party was an analogy to this and that is the bar code you see in a grocery store. So the grocery store is a bar code that doesn't necessarily use color but it uses the thickness of a black line. So you’ve got a black thick line and then a thin white line and another black line and the pattern of those lines is kind of the grocery store's version of the heat map. It's a pattern that the computer recognizes as a particular product. And as I presented there, I showed two bar codes that look very similar but they have some differences. And the reason they look similar is because both bar codes indicated that it was a loaf of bread. The reason they were different is because one loaf of bread was wheat bread and one loaf of bread was white bread. What the computer was able to do is it was able to scan the bar code and there was a program in the computer that said that one's a wheat bread and that one's a white bread. Charge him $1.25. Well, imagine we now have a bar code that is unique to every individual patient and that's this heat map. And if we can correlate that bar code with an outcome, when you now scan a bar code into the computer, it might say based on this bar code, this person has this type of tumor that will respond to this drug, give them Velcade. So the goal here is very similar to the grocery store is to develop these visual bar codes for each individual tumor and that pattern is then read by a computer scanner just like it is at a grocery store. That goes into a computer, the computer has a program that recognizes that signature as a responder or a non-responder and then spits out a decision support that says these are the actions you might consider taking based on my reading of that heat map or in this case, bar code.
Jenny: Okay. Well, we all want to be scanned, I think.
Dr. Van Ness: Yes. And I have to admit, the danger I got into at the Beach Party, was that as I explained this I think I was a little overly enthusiastic and I sold it a little too well and every patient then started lining up and said I want my bar code scanned into the computer. I had to back off a little bit and say that is the ultimate goal of all of this. We need to generate the bar codes, we need to associate them with an outcome and we need to develop the computer programs that have already been developed in the grocery store but we need to develop them within clinical applications. So we do have a few steps we have to go through before everybody lines up for their bar code.
Jenny: Okay, let me ask you some follow up questions. Instead of looking at these three billion things of DNA in a patient, you are typically looking for the genes that that DNA then expresses. So you're looking for target genes that could be wayward genes that are not acting normally. So now you have a subset of list and then those genes are creating proteins that sit on top of the myeloma cells. So some of these myeloma therapies are targeting a certain protein per se, that's when you kind of get to this actual drug that's being developed or whatnot. But if you're looking at this gene expression and there's a test that is kind of like maybe a part of the scanner, the gene expression profile test, is that what that test does? I think people sometimes understand that test and some don't and most don't get it at all and very few myeloma patients really understand the biology of their disease. Do you want to address that gene expression profile test and if that could be part of that scan that we do and do today?
Dr. Van Ness: So I'm going to give you the good news and the bad news. Let's start off with the bad news and I put that sort of tongue-in-cheek. If I get the expression, in our case we have about 23 genes. And these 23 genes have a pattern of expression that is different in a patient that responds to Velcade versus one that doesn't. And so if I now develop that as a bar code reader where there are reds and greens in there and every patient is now red, the computer might be able to say based on that pattern, you may be a responder or a non-responder and that's useful information. But the bad news is I don't necessarily understand the biology of why those 23 genes are as predictive as they are. That's going to take another set of research questions to ask, why did those genes come up? And there are two questions that one might ask that are really interesting. One is: of those 23 genes, are there any in there that can help explain functionally why the person's not responding to the tumor? I just simply said I have a signature that says you will or won't respond. The second question is how do those gene expression patterns identify that, what's the functional component of that? That's another interesting and important research question. The next research question that could really be interesting and important is, if I understand some characteristic of those particular genes, could they direct me to develop new therapies based on that particular set of genes and that's another really interesting question that needs to be addressed. In this particular case, we're still at the point of having 23 genes. They seem to do a pretty good job of predicting a response but to be honest with you, we really don't understand how those 23 gene expression patterns all interact to give us that prediction. Now I would venture to say that the person scanning the bar code in the grocery store, if you handed them that bar code without the scanner and the computer and asked them to tell you what it is, they couldn't do it. They'll say "It's a bar code. I don't know." But once you scan it, the computer says it's wheat bread. Nobody understood the pattern but the computer understood the pattern enough to identify it as wheat bread. So the analogy here is we have identifiers, they may actually be clinically useful identifiers but we still have another level of research to understand the function of those identifiers and whether those identified genes actually could be really effective new targets for new therapies.
Jenny: Okay. So I understand what you're saying. You're saying sometimes it's a little too early to have that information because you're still trying to divvy people in the buckets between who responds and who doesn't. So if everybody is marked with these genetic features and the technology isn't there, the research isn't there yet to know what to do with the why or why it's happening, then if it's present, if those genetic markers can only be found like at diagnosis or at relapse, wouldn't it be better if everyone got it regardless, just in preparation for the development that will come later with what to do with that information? Does that make sense?
Dr. Van Ness: It really does. There's something to be said for everybody having a genome done and having an expression pattern done so that it's in the bank and then as we understand more, you can go back to that genome or back to that expression pattern and learn things that are useful for that particular patient that maybe you didn't know on January 2015 but in January 2016 you now have the capability to go back to the data and say well, I know more now. Now I can go back to that database and now get more information for that particular patient. So I do agree with you that if we had unlimited resources, I would argue that every patient should be sequenced and the profile should be done in every patient because ultimately I think more and more information is going to make that useful.
Jenny: So are you doing this with the RNA sequencing or like a whole genome or whole exome sequencing process or using this gene expression to the GEP70 or what are you using to sequence these genes?
Dr. Van Ness: We're actually looking at every gene so we're using what's called an Illumina platform that looks at every gene so it's going to assess every gene that's expressed. But we're well aware of the GEP70 and we can subset so that gives us the opportunity if we assess every gene as a new signature becomes available, we have the data that we can now query that data with the GEP70 profile for example. So you bring it back to the question you asked which is we can take advantage of the subsets that other people have generated to query the data we have because we've made the decision to be all-encompassing and to maintain the database on all of the genes being expressed not just the ones we think are important today. So it does give us a very valuable resource to go back to.
Jenny: Well, I guess I just don't understand… sometimes some of the doctors will say well, we can't really use that information in the clinic yet but to me that doesn't make sense. Because things are going so fast and the development is happening so quickly that you could go retrospectively and say okay, let me find all these patients that have these five genes together or out of the 23 or whatever, and oh, those were the ones who didn't respond to transplant or whatever.
Dr. Van Ness: Right. So I'm going to give you what's now become kind of a new difficult challenge. In the past the technology challenge was, can you generate a technology that is relatively inexpensive and relatively quick to generate an entire genome or an entire expression profile. And the answer today is that's pretty doable but now we have a new challenge. And the new challenge is that has generated these enormous data sets but now you've got three billion base pairs to wade through to try to figure out which ones are the important ones. And so the people who are going to say what's on this are going to be the computer programmers. So some of my best friends and I mean this sort of the people that I like to talk to from my research components are computer science guys. These are guys that don't necessarily understand the genetics and the biology but they really understand complex data sets and they understand the need to search these data sets and find patterns. And so these are the people that we spend a lot of our time with now is trying to generate new… and there's a term that's commonly used, it's called machine learning. And in fact, the way some of these computer programs work is that they go into a data set -- now I'll give you an example. And they'll say let's pick ten random genes and ask how well they are associated with this response. Well, if you pick ten random genes the chance they're going to have anything to do with it is really small. So the computer says that didn't work, let's try another ten. And the computer says that didn't work, let's put another ten. Well that worked a little bit but let's pick another ten. Oops, that works better, let's keep those and add ten more. And what happens is the computer, because it's a computer, can go through these multiple testing iterations over and over and over again millions and millions of times in a way to try to select the best set of genes that might give you the predictor. And this is what machine learning is, it's the computer learning as it goes. As it’s going through the database, it's constantly refining, refining the database to keep getting better and better predictions. Some of the programs we run to try to get our signatures, we have at the University of Minnesota what's called the super computer. These are some of the fastest computers in the country in terms of their ability to process large, complex data sets. Some of the programs we run to ask the computer can you identify a subset of signatures can take four or five days to run, just the constant run and hum of the computer running all of these possibilities through to try to identify the best group of signatures. This is where I think the next challenge is. It's now having the ability to run these large data sets from patients into these computer programs that give you accurate, predictive capabilities. And a lot of these programs are still being developed, it is a real challenge. It also means that this becomes interdisciplinary. I have no ability to program a computer but I do have an ability to pick up the telephone and call somebody that knows how to do that. And so what I think is happening in the field of genetics and genomics, it's becoming much more interdisciplinary, we are bringing people in who have clinical expertise, research expertise, genetic expertise, computational expertise, all of those people are now having to work together because no individual has all the expertise to really make an impact on this. So I think that's another reason why the Mayo-University partnership has been working is because we brought between the University and Mayo Clinic, we have all those areas of expertise involved in this project.
Jenny: Absolutely. It sounds like it's going to require that team effort. I have several other questions, but I want to allow enough time for caller questions as well. I saw that recently, maybe you can address this quick question that 11;14 is typically thought of as a low-risk profile in myeloma and I saw something that said it might not be the case. Did you hear the same thing and how could you address that?
Dr. Van Ness: I did and I'm going to blank on there is a genetic component. So again, it comes down to 11;14 is one genetic hallmark of a particular tumor. That doesn't mean that there aren't other genetic events going on in everybody's tumor. So it becomes a little bit dangerous to say all 11;14s are going to behave the same way because in addition to 11;14 there are other genetic events that are occurring in different people's tumors. As I recall and I saw this announcement that the investigators identified another genetic abnormality that was in a subset of patients with 11;14 that actually put them into a higher risk category. And to be honest with you, that's really not surprising. It's not that this was unimportant, don't get me wrong, it's a very important observation, but it's not surprising that as we know more and more about the genetics, each individual is going to have a group of genetic events that are occurring that are going to impact their risk for disease progression or response. So an 11;14 in general on average is a low-risk group. How many people in the population of patients are sitting smack dab in average, that there's variation on either side of that characteristic. And I think what these investigators were able to do is to identify another subgroup within that 11;14 that actually had some other characteristics that put them in a higher risk.
Jenny: Well, that makes a lot of sense. So I have one question that I'll ask before I open it up to caller questions. Based on how many we have, I have other questions. But what can patients do to help you cycle faster with what you are trying to learn? Because it sounds like you're at the beginning of your study, you're going to learn some very critical things about who responds and who doesn't to these proteasome inhibitors and the different types, maybe one is better than the other, maybe it's not, maybe they're all the same and they respond the same. But what can patients do to help accelerate this type of learning?
Dr. Van Ness: I think the most important thing patients can do is to participate in research studies and critical trials. I mean that becomes for a lot of critical trials -- again I'm going to talk in generalities but if you look at all cancer patients across the country, about 5% of cancer patients are on clinical trials.
Jenny: Kind of sad and pathetic, in my opinion.
Dr. Van Ness: Yes. So it's an untapped resource and I think there are concerns about the ethics of genetics and the privacy issues and confidentiality issues. I think that precision medicine is going to have to allow people to provide their samples, allow researchers to identify all of these genetic components with faith in the system that we're not all going to go running out and breach confidentiality and sell these things to insurance companies. That kind of breach of confidentiality really hasn't happened. And so I think patients have some concerns about whether the genetic information that they may be revealing could come back to harm them or people in their family. And I think we have a number of protections in place to try to do everything we can to maintain that confidentiality and I think it works pretty well. So I think patients should be willing to participate in donating samples and their clinical information that's associated with that. And the other part of precision medicine, there is a component of personalized medicine that I take very seriously and I promote very seriously. Personalized medicine also means personal responsibility and participation. And that is as we start off our conversation at the very beginning, as patients learn more about their own unique characteristics, they should help and participate in their therapeutic approaches. Patients are becoming very smart about this kind of stuff and I do think personal involvement and personal responsibility in managing their particular treatment becomes very important. And I know on one hand physicians get a little nervous if patients are trying to tell physicians what to do. But I also think that physicians in general and particularly people like my colleagues at the Mayo Clinic, they really do appreciate the fact that the patients are actively involved in the decisions that are needed to be made based on their own personal characteristics. And I think most physicians, the good ones, embrace that.
Jenny: Well, I think I want to get on my soapbox for a second because when it comes to patient data like donating your samples or donating patient-reported outcomes or something, I think if you're in a situation like diabetes, you might be concerned about patient privacy but if you have a disease that you're going to die from and in short order, I don't think any of that really matters. To me personally, it doesn't matter. You want to know what I have for breakfast? Okay. If it's going to save my life, I don't care. And then I do believe that if patients -- I agree, completely, because if patients took it upon themselves to say "I could speed up or double or triple the time to answers because I'm either participating in a clinical trial or because I'm donating a sample or because I even ask intelligent questions about my own type of myeloma." That's a direct relationship between activity that patients can take and their clinical outcomes. So I think we have the most to win or lose from this that we should be involved so I completely agree with your personal responsibility comment. Let me open it up for caller questions. If you have a caller question for Dr. Van Ness, please dial 347-637-2631 and press 1 on your keypad.
Caller: This is Eric. Great, thank you very much. It sounds as if your research and other research to date has shown that there's extreme instability in a person's genomics. And I'm wondering if we all got baseline sequencing done and banked today, that first relapse or post-transplant, all these other events in myeloma history, would we need another sequence to make sure that we can go from being a secretor to a non-secretor or get a different genetic abnormality in the process?
Dr. Van Ness: I think that's a great question. So the question is if you get a sequence at diagnosis and you can learn things from that and then maybe two years later a relapse occurs, would it be appropriate to do another sequence. I think the answer is yes. I think if you can learn something about the individual in terms of how the genetics may have changed in that tumor and the more you do of that, the more you start learning of the potential for myeloma to make these kinds of changes, that will be beneficial to other people as well. So I would argue that oftentimes to me, at least in my research, one of the most valuable samples and one of the most difficult ones to get is the relapsed sample because I really do want to reprofile the relapse sample and ask what changed. I can make those changes in the laboratory, I can take a myeloma cell in the laboratory and treat it with a drug and in three months I can get a resistant population that grows out and I can characterize it. But the real test is going to be can I characterize a relapsed patient sample and learn something? Because to some extent, if you relapse with drug resistance, for me to give you an answer that says "Based on the profile of the relapse, you're going to be resistant to the drug." That's not novel information, you already know that, that's why you're in this kind of relapse. But the profile on that relapse sample may actually inform the clinician what might be the next best therapy to treat that particular relapse. So I think the relapse samples, recurring samples and retesting them can be incredibly valuable.
Caller: Thank you very much.
Jenny: Thank you, Eric.
Caller: Thank you for taking my call. Thank you, Doctor, for being on the show with us this morning. It's been really interesting and I've learned so much. I want to understand more about the proteasome inhibitors, how can you translate that work into research into other drugs?
Dr. Van Ness: Good question. So we're using the proteasome inhibitors as an example of a profile that might predict response to those proteasome inhibitors. But remember, we're going to have that profile. So if we have a full gene expression profile and now we apply it to patients receiving Thalidomide or Pomalidomide or some of the new HDAC inhibitors, some of the new drugs, we can do exactly the same thing. We can say is there a set of genes that might predict whether you're going to respond to that drug that may be well different than the set of genes that predict your proteasome inhibitor response. But the database is available to ask that question so that's why we're really interested in getting a comprehensive database at the very beginning so that we can now use this to potentially predict the multitude of different therapeutic responses. We're not there yet but that's the goal.
Caller: Excellent. I also like your comment that it's really important that patients start becoming very involved in their own care. So thank you.
Jenny: Thank you so much. Dr. Van Ness, we're out of time but I have one final question. How do you go about housing the data once you've gathered the data?
Dr. Van Ness: That's one of those things that my computer people are in charge of. In other words, you generate these spreadsheets and data files that can be accessed and you put up firewalls that people can't get accessed to unless it's password-protected. So it really does become these very large within the computer data sheets of this kind of information. And you can imagine if you're looking at 20,000 genes in one sample, you've got a lot of file entries in there. Fortunately, computer programs are available to house that kind of data and store it for us so that we can pull it back up. And I must admit there are people that I have working with me that are much better at developing those databases and then recalling those databases that I am. That's part of the interdisciplinary need for this project.
Jenny: Correct, I would think so. Dr. Van Ness, thank you so much for taking the time. I think the work you're doing is completely fascinating and could give us so many clues into what we do with certain drugs and how each patient gets treated. So thank you for dedicating your life to this work. It's truly amazing that there are people as smart as you out there.
Dr. Van Ness: You're very welcome and thank you for the opportunity to have this discussion. It's always a good thing to do to talk with people and talk to patients about what's going on. I think it's part of our obligation to make sure that the patient groups are well informed about what's going on and as a community, the myeloma patient groups I find to be just amazing people with incredibly positive outlook and I always get charged whenever I talk to patient support groups.
Jenny: Well, thank you for helping us to come to conclusions faster. We are very excited to hear about your results and we'll be watching for them. Thank you so much. Thank you for listening to Myeloma Crowd Radio. We believe that patients can help support the discovery of a cure and we encourage you to become involved.
Trending Articles
Have Any Questions?
Thank you for your interest in the event. If you have any questions, we would love to help!
Feel free to give us a call or send us a message below.
Get In Touch With Us
1-800-709-1113
Support@healthtree.org
More Podcast Episodes
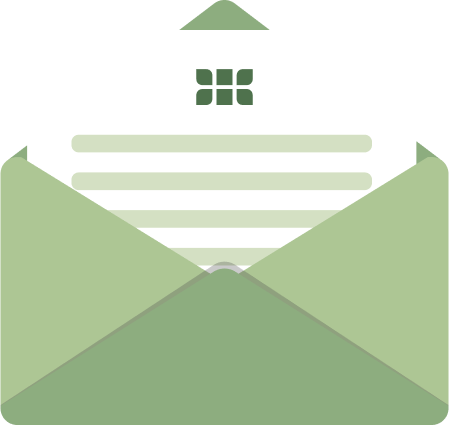
Get the Latest Multiple Myeloma Updates, Delivered to You.
By subscribing to the HealthTree newsletter, you'll receive the latest research, treatment updates, and expert insights to help you navigate your health.