Evaluating Racial Influence on Machine Learning Predictive Models
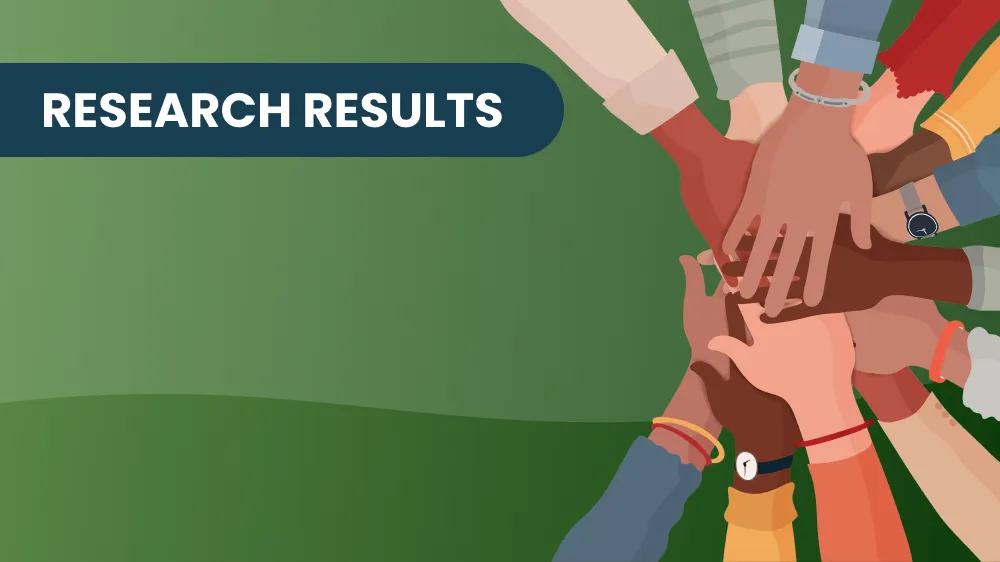
Artificial intelligence and machine learning open new doors of possibility for myeloma research, but can factors like race affect AI-based predictive models?
In a prior article, HealthTree shared Machine Learning research performed at multiple academic centers to predict when a patient’s monoclonal protein may increase or decrease, even before lab results are returned to the patient during the 3-7 day waiting period.
Why Racial Equity Matters in Predictive Models
Treatment decisions often need to be made quickly, so using unbiased predictive models that span across various racial groups is essential. This study explored the role of race in predicting monoclonal protein (M-protein) levels using machine learning techniques.
As a reminder from the prior article,
The three most important factors for an accurate M-protein prediction were serum total protein and the previous two M-spike values.
To analyze the data to include race, two models were developed based on the random forest algorithm:
-
Model A: Incorporated prior M-spike values, total serum protein, race, and ethnicity.
-
Model B: Included all variables from Model A along with additional biomarkers such as immunoglobulin levels, height, weight, and albumin levels.
The goal was to determine whether including race improved the model’s predictive power.
Study Design: Testing the Role of Race on Model Performance
A total of 619 patient-based observations were analyzed, with 80% used for model training and 20% for validation.
For patients with available race data, the first dataset was made up of 90% Non-Hispanic White, 6% African American and 4% other racial groups. The data set was then adjusted to decrease the Non-Hispanic White patients to 78%, with the percentage of African Americans and Hispanic patients increasing.
The predictive models showed minimal differences when race was included or excluded:
-
Model A: Excluding race resulted in an insignificant improvement. (Excluding race resulted in a root mean squared error (RMSE) of 0.2634 and an R² of 0.7440, while including race yielded an RMSE of 0.2631 and an R² of 0.7445).
-
Model B: Including race slightly decreased predictive performance. (Excluding race led to an RMSE of 0.2524 and an R² of 0.7670, while including race led to an RMSE = 0.2555, R² = 0.7604).
-
Even with the additional racially diverse dataset, race had minimal impact on predictive accuracy
Results
Researchers concluded by stating that race did not significantly enhance or harm this Machine Learning model’s performance.
Future AI-based studies should test their models in racially diverse groups as patients’ myeloma can behave differently across races. Future research should take into account the importance of genetics, socioeconomic factors and prior treatments to address broader disparities in myeloma care.
The development of unbiased predictive tools will lead to more equitable care for multiple myeloma patients.
To continue reading about research being conducted at HealthTree, follow the link below:
Source:
Machine Learning in Myeloma: Do Racial Differences Influences Systemic Impact of Multiple Myeloma?
Artificial intelligence and machine learning open new doors of possibility for myeloma research, but can factors like race affect AI-based predictive models?
In a prior article, HealthTree shared Machine Learning research performed at multiple academic centers to predict when a patient’s monoclonal protein may increase or decrease, even before lab results are returned to the patient during the 3-7 day waiting period.
Why Racial Equity Matters in Predictive Models
Treatment decisions often need to be made quickly, so using unbiased predictive models that span across various racial groups is essential. This study explored the role of race in predicting monoclonal protein (M-protein) levels using machine learning techniques.
As a reminder from the prior article,
The three most important factors for an accurate M-protein prediction were serum total protein and the previous two M-spike values.
To analyze the data to include race, two models were developed based on the random forest algorithm:
-
Model A: Incorporated prior M-spike values, total serum protein, race, and ethnicity.
-
Model B: Included all variables from Model A along with additional biomarkers such as immunoglobulin levels, height, weight, and albumin levels.
The goal was to determine whether including race improved the model’s predictive power.
Study Design: Testing the Role of Race on Model Performance
A total of 619 patient-based observations were analyzed, with 80% used for model training and 20% for validation.
For patients with available race data, the first dataset was made up of 90% Non-Hispanic White, 6% African American and 4% other racial groups. The data set was then adjusted to decrease the Non-Hispanic White patients to 78%, with the percentage of African Americans and Hispanic patients increasing.
The predictive models showed minimal differences when race was included or excluded:
-
Model A: Excluding race resulted in an insignificant improvement. (Excluding race resulted in a root mean squared error (RMSE) of 0.2634 and an R² of 0.7440, while including race yielded an RMSE of 0.2631 and an R² of 0.7445).
-
Model B: Including race slightly decreased predictive performance. (Excluding race led to an RMSE of 0.2524 and an R² of 0.7670, while including race led to an RMSE = 0.2555, R² = 0.7604).
-
Even with the additional racially diverse dataset, race had minimal impact on predictive accuracy
Results
Researchers concluded by stating that race did not significantly enhance or harm this Machine Learning model’s performance.
Future AI-based studies should test their models in racially diverse groups as patients’ myeloma can behave differently across races. Future research should take into account the importance of genetics, socioeconomic factors and prior treatments to address broader disparities in myeloma care.
The development of unbiased predictive tools will lead to more equitable care for multiple myeloma patients.
To continue reading about research being conducted at HealthTree, follow the link below:
Source:
Machine Learning in Myeloma: Do Racial Differences Influences Systemic Impact of Multiple Myeloma?

about the author
Jennifer Ahlstrom
Myeloma survivor, patient advocate, wife, mom of 6. Believer that patients can contribute to cures by joining HealthTree Cure Hub and joining clinical research. Founder and CEO of HealthTree Foundation.
More on HealthTree Research
Trending Articles
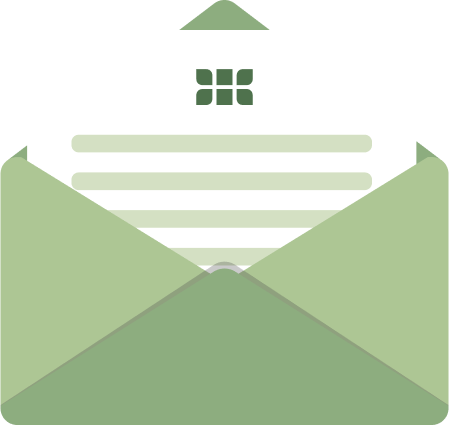
Get the Latest Blood Cancer Updates, Delivered to You.
By subscribing to the HealthTree newsletter, you'll receive the latest research, treatment updates, and expert insights to help you navigate your health.